Using Artificial Intelligence in Pharmacovigilance
October 12, 2021
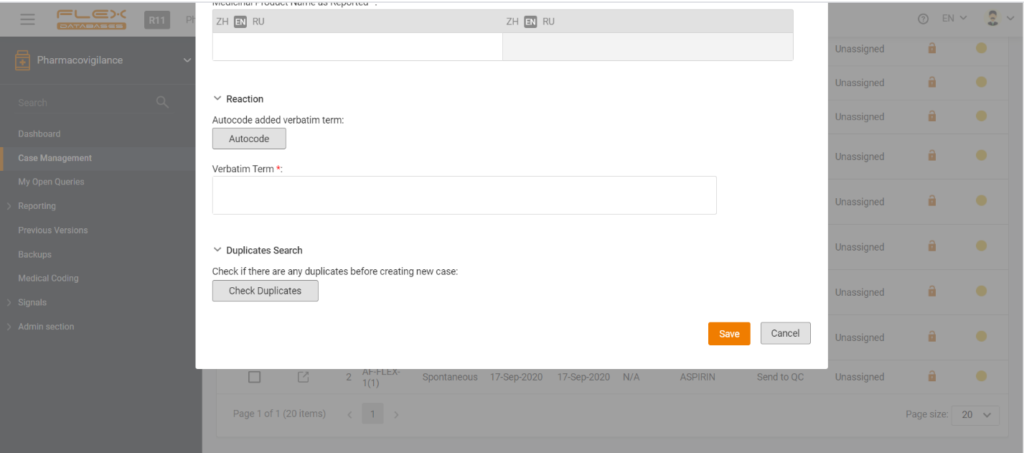
As we briefly discussed in the previous chapter, Artificial Intelligence (AI) is used to simulate the processes of a human brain with the help of computer systems. It encompasses various technologies, including rule-following, reasoning – using rules to reach rough or specific conclusions, learning, and self-correction.
Implementation areas of AI
In the area of pharmacovigilance electronic systems, AI technologies could be implemented in case collection, consolidated reporting, and signal and quality management.
For example, using machine learning algorithms to automate medical coding according to MedDRA dictionary for medical terms or WHODrug dictionary for medical products.
The image below demonstrates how AI based on a neural network is used to detect and manage signals, find duplicates, and auto-code in the Flex Databases Pharmacovigilance system.
Additionally, AI is used in safety signal detection, including:
- multimodal signal detection,
- signal detection using neural networks,
- predictive signal detection.
In general, AI and other automation technologies in pharmacovigilance allow to eliminate human errors, standardize processes, shorten the processing cycle, and reduce manual work.
Examples of AI technologies
Technology | Short description | Use cases of pharmacovigilance electronic system | |
Machine learning (ML) | Machine learning (ML) is a subset of AI, allowing the creation of algorithms that can learn and make predictions based on the data. Instead of following a predefined set of rules and instructions, these algorithms are taught to identify patterns in big data and improve over time and with more data. This technology helps identify potential signals. | Case collection, periodic reporting, signal management, and risk management. | |
Neural network | System replicating a neural structure of a mammal’s brain. Neural networks usually consist of multiple layers which are formed by numerous nodes. This technology helps identify potential signals. | Case collection, periodic reporting, signal management, and risk management. | |
Semantic search | Semantic search is meant to improve search accuracy and deliver relevant results by understanding user intentions and search query context. | Case collection, periodic reporting, signal management, and risk management. |
AI use cases
Use case 1. Automated case collection and evaluation of serious adverse events (SAE) from online comments on Indian websites.
Traditional safety data collection is challenging in this area, however, using of artificial neural networks and sentiment analysis allows to automatically identify characterizations of the researched objects in the emotionally coloured text.
Use case 2. Predicting the safety profile of a developed drug
Researchers combined in vitro data with safety information from FAERS database, and created machine learning models, allowing to predict future adverse events based on the data point from other databases, such as PubMed.